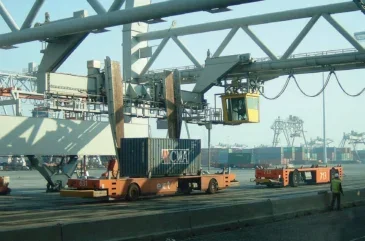
AI is coming. It is coming to a hoist near you. It will make your lifting more efficient.
But it won’t just be your lifting that it will look at. Lifting is part of material handling. AI will consider the whole of your material handling and will adjust your lifting to make that more efficient too.
And it won’t just be material handling. Material handling is part of production. AI will look at your production and will control your material handling (and so your lifting) to make your production more efficient as well.
And it might not be just production. Production depends on the supply chain – to enable you to produce in the first place – and on the demand for your product – which will determine how much of it you want or need to produce at all.
AI can consider factors that affect supply chains. And to predict – or, until now, to form some sort of semi-accurate guess at – what demand is likely to be, you need to know what the economy is doing now, and what it will be doing six weeks or six months or six years from now.
If you are producing products for new houses you have to know what interest rates are going to do, now and in the short term and in the long term; or if you are producing textiles or clothing you need to know if some teenage influencer with half a million followers is going to decree that pink is no longer the right colour to wear but purple with blue (but not green) spots is what everyone has to be seen in this spring; or if you are producing ice-cream, you need to know whether next summer is going to be hot or miserable or next to non-existent; and whether chocolate or pistachio will be the preferred flavour.
AI will – or can, or could – examine vast amounts of data about all of those things, and will – or can or could – come up with conclusions about them and decisions about them that might, among other things, tell your hoist whether to lift two bundles of pink cloth and one of purple and blue this workshift, or the other way round. Or in your ice-cream factory it might tell your hoist to lift and carry loads and loads of chocolate flavouring, but to hold the pistachio – in fact to carry those nuts to the waste bin.
In other words, AI can analyse incredible amounts of data, about almost anything, and come up with conclusions.
Don’t ask me how it comes up with those conclusions. In fact, don’t ask anyone that question because nobody on this planet knows the answer. And that is because the whole point of AI is that it teaches itself how to do things, and it doesn’t share that knowledge with us; we are its mere human sidekicks who devised AI in the first place. All that AI demands of us is data, and not just data but lots and lots and lots and lots of data. Give it data, and tell it your problem, and it will tell you what you should do.
They call it Machine Learning, or Deep Learning, and it is probably what distinguishes AI from mere smart technology. (Academic definitions get hazy in this area.) A competent human programmer can write an algorithm to tell a hoist the most space-efficient way to stack rolls of cloth. That is a relatively simple problem. A hoist that is part of an AI-connected factory can tell what it the most space-efficient way to stack rolls of cloth given that the purple ones are going to be wanted most; and it is far from impossible that in the very near future it will also be monitoring vast chunks of the teenage social media to decide whether or not purple is indeed likely to be wanted most. That is a hugely complicated problem that no human programmer could attempt. Complex problems with lots of contributing factors are what AI is good at.
Big Data is a necessity for it. The data can come from traditional sensors that measure simple things like speed or distance, or from cameras, microphones, lidar, sonar or anything else that gives any kind information about the world. Computer vision uses AI: video cameras that recognise faces, or ships at sea, or cloud formations that are relevant to the weather, or, on a self-driving car, whether the traffic light ahead is green or read and whether or not it applies to the lane of traffic that you happen to be in at the time – all of these are current applications of Artificial Intelligence.
So, too, is predictive maintenance. Programmers can write algorithms to predict when a brake lining will need changing, but AI does it better. It can take many more parameters into account. Most manufacturers, and all but the most basic hoist installations, now include predictive maintenance as more or less standard – and most of the programming for them has involved AI.
Thus at Siemens, Artificial Intelligence technology and data analytics are used to predict when equipment is likely to fail before the breakdown actually occurs. Maintenance schedules are updated based on these forecasts.
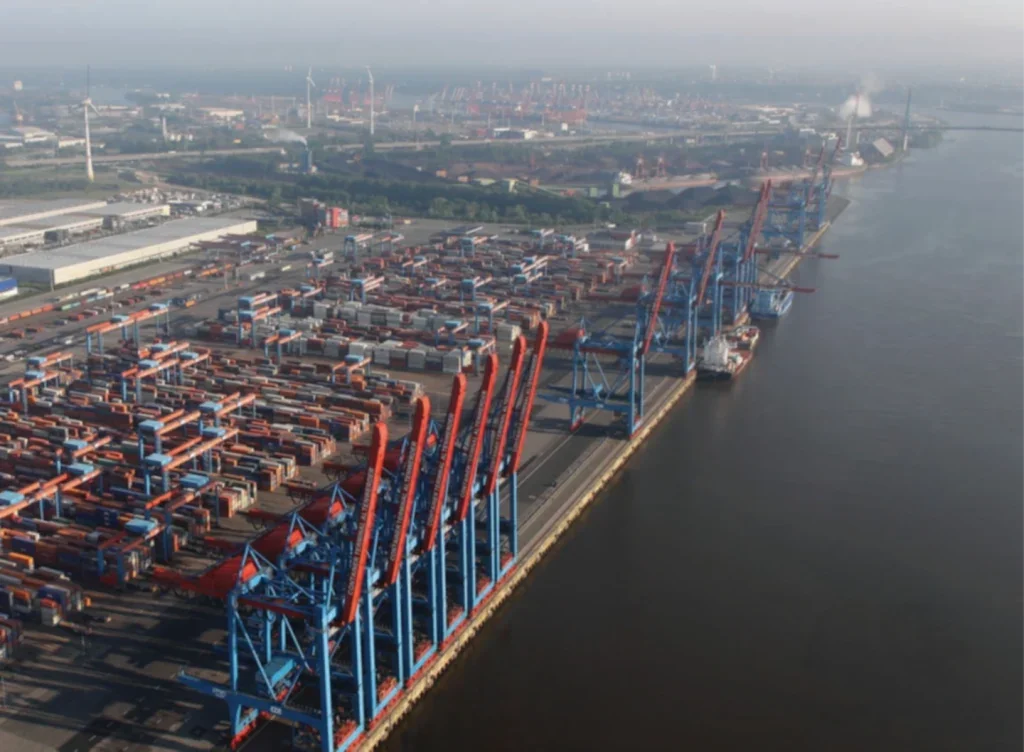
“Incorporating AI on crane maintenance activities results in an increase in uptime and a decrease in repair costs, extended life cycles and savings in time and money on emergency repairs” says the company. “Crane data is the source for this. Ports and terminals are generating large amounts of data coming from different types of sensors – the crane management system, the maintenance system and any other available source. For predictive maintenance these sources are combined to generate algorithms that predict when which failure is likely to occur.”
The AI technology behind this solution can be split into two main categories, say Siemens. So-called Supervised Learning is for regularly occurring events like brake wear and rope stretch. The network learns from historical patterns in the data, applies them to the current data, and predicts which failures are likely to occur within a given time frame.
Unsupervised Learning predicts rarely occurring events such as hoist gearbox failures. Here, the neural network learns the pattern of a normal state of equipment, compares the current data to it, and sends out an alarm whenever the data deviates from normal.
Many AI systems are so complex that their designers cannot explain how they reach their decisions. This is particularly the case where there are a large amount of non-linear relationships between inputs and outputs. The most widely used current textbook on the subject, by Stuart Russel and Peter Norvig, says, quite simply, “The reason that deep learning performs so well in so many applications is not known.”
AI is autonomous – it doesn’t need people – and adaptable – it can cope with the unexpected, with events that no-one had even considered likely enough to put into a human-written software program. Operations research, planning – especially complex planning where hundreds of factors are relevant and not all of them are known, so having to deal with probabilities – are therefore the sort of problems which seem ideal for it. The more variables, the more uncertainties, involved, the more AI becomes useful. The lifting application which probably contains as many variables and uncertainties as any other is the container terminal. It is therefore not surprising that this is an area where AI is making inroads.
At a container terminal there are thousands of containers, hundreds of trucks to be loaded, dozens of ships to be unloaded, many vehicles, many port cranes and gantry cranes and reachstackers. Ships arrive not always to schedule but affected by winds, tides and weather. Containers need to be unloaded, stacked efficiently – storage space is always at a premium in terminals – then transferred to the trucks, but not necessarily in the order in which they were unloaded. Immediately needed containers should be in a top-row stack, not stuck on the bottom with four others above it. And the arriving stream of trucks and truck-drivers needs to be loaded up with correct container as promptly as possible, because waiting makes traffic jams which make more delays, and costs money.
Each of these demands are well suited to AI solutions. All of those demands taken together are even more well-suited to AI. But AI is a work in progress; all of the above is being implemented, but in stages.
Thus in March 2024 Kalmar and Forterra announced a partnership to develop an autonomous (ie self-driving) terminal tractor solution for container terminals, distribution centres and industrial yards. Self-driving cars of course depend on AI, have been almost with us for several years, and in a few cities are in actual operation. Self-driving straddle carriers, however, are already in widespread operation in container ports and have been for some time. One reason that straddle carriers have arrived before terminal tractors is that their challenge is simpler. Straddle carriers operate in fenced-off areas, free of pedestrians and, generally, therefore, much more predictable and with less disastrous consequences of failure. Terminal tractors, however, are also needed, and they must be able to operate additionally in mixed traffic, where external vehicles – trucks and the like – and pedestrians are encountered. Their AI solutions therefore need therefore to be much more sophisticated and robust.
Forterra is one of the foremost innovators in the field of driverless technology and its AutoDrive system is one of the leading driverless systems for complex conditions including ports and logistics centres.
In the joint development initiative, Kalmar will supply autonomous terminal tractors equipped with the drive-by-wire and hardware kits required for autonomous operation. Forterra will supply its AutoDrive autonomous driving system and related tools, while Kalmar will integrate these with its Kalmar One automation system. Sales and deliveries of the solutions will be done by Kalmar.
The development will proceed through several steps. Currently Kalmar and Forterra are carrying out one to two-month demonstrations at customer sites with autonomous terminal tractors with safety requirements adapted for each location. While some locations require a safety driver, known as “Driver In”, some demos will operate without a safety driver – that is, “Driver Out”. This enables terminals to gain an understanding of the performance and reliability of autonomous driving technology in their own working environment.
At the same time, drive-by-wire development will continue for the Kalmar terminal tractor platform required for the autonomous solution. The proof of concept for Forterra AutoDrive and Kalmar One integration will be completed in early 2025.
The first goal, of being able to provide autonomous terminal tractor capability without safety drivers in closed-off automated areas, is expected to be attained by mid-2025. “This will be followed by environments allowing interaction with trucks whose drivers have received the appropriate induction training, and finally by full mixed-traffic operation,” says Timo Alho, head of the product management in Kalmar’s Automation and Projects Division.
“Every terminal is different” says Mike McGhan, head of Commercial Logistics, Forterra, “and we are currently working closely with a wide range of our customers to both ensure safe operations and help them discover the best value from automation for their individual business. “Our goal in this partnership is to develop a true integrated system that provides the customer with a single point of contact for systems, safety and performance optimisation, as well as scalability to fullsize logistics operations.”
The main markets for the solution are North America, Europe and Oceania. The autonomous solution will be available for the Ottawa T2 and Kalmar T2i terminal tractor models, as well as the respective battery-powered models once they are on the market.
Dr. Nils Kemme is managing director at HPC Hamburg Port Consulting, which operates the adjacent Altenwerder and Burchardkai terminals. “At Burchardkai we are testing the use of predictive maintenance to predict the remaining service life of cables on the container gantry cranes,” he says.
“At Altenwerder, algorithms are used. In principle, these make all decisions relating to the automated equipment. For example: which container is to be placed at which storage space, which automated transport vehicle is to transport which container from A to B, which route is to be taken? At this terminal, all these decisions are made by algorithms. These are rule-based algorithms, but in a broader sense, they can also be regarded as artificial intelligence.
“The greatest challenges involving artificial intelligence in logistics are about data availability” he adds. “The data needed to train the AI is usually available somewhere at the terminal. The way in which the algorithm used by the AI is trained forms the basis for its subsequent application, which means that the more data that is available, the better the artificial intelligence will function. However, it is often not possible to locate this data, and many systems must first be combined with one another to allow this to happen. This represents the bulk of the work involved in developing functional applications of artificial intelligence for terminals.”
One source of such data is drones. They can of course view an entire port area, landside and the maritime approaches; and send real-time information about it. In May 2023, the Port of Antwerp-Bruges introduced its ‘drone in a box’ network. Called D-Hive, it comprises six autonomous drones that cover an area of over 120 square kilometres, giving monitoring capabilities throughout the Antwerp port region.
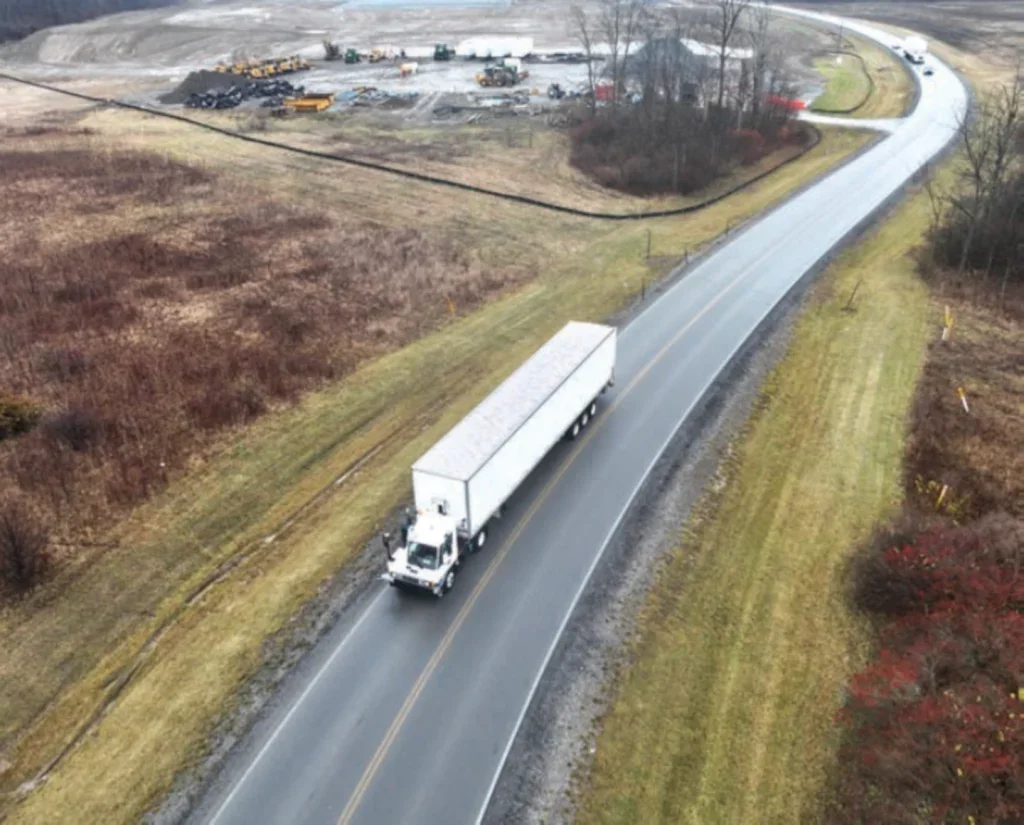
The drones play a crucial role in tasks such as berth management – they can see exactly where vessels are at each moment – infrastructure inspections, monitoring operations, detecting oil spills and floating waste, as well as supporting security partners during incidents.
By harnessing the data from these drones, the Port of Antwerp-Bruges aims to enhance operational efficiency, safety, and environmental monitoring, while also facilitating prompt response and decisionmaking in critical situations.
We have said that machine learning is crucial to AI. One way that machines learn is through trial and error. The software takes a situation, changes a parameter, and if that change improves the situation, it is kept; it if does not, it is discarded. Repeat the process several million times, changing as many parameters as exist, and eventually one of the solutions will be optimal. (If it sounds crude, it is not. It has, after all, produced human beings. Evolution is simply biology applying trial and error.)
But AI cannot apply trial and error in the real world; an error that, for example, sends a fully loaded supertanker crashing into a dockside crane would hardly be acceptable. The solution is a digital twin: a simulation of the port, fed by the vast streams of data that we have been talking about, where experiments in their millions can be simulated and the results observed. The best results can then be applied to the real port.
The digital twin is a useful tool for AI, but not only for AI. Real-time data on weather conditions, tidal movements, and vessel traffic can be integrated into it, enabling safer navigation and docking procedures for vessels and giving port operators the clearest possible overview of their infrastructure and operations. Optimizing vessel berthing schedules to minimize fuel consumption and emissions is just one way that AI and a digital twin can feed off each other.
Hence the Port of Rotterdam’s Digital Twin project. Rotterdam’s Port Authority’s aims to have fully autonomous shipping within it by the year 2030. The development of the port’s digital twin is, they say, an important step in the process. This digital Rotterdam incorporates data about all infrastructure objects, shipping movements, weather conditions and hydrological readings. It is a long-term project: the first phase got underway in 2018 with the development of an IoT (Internet of Things) cloud-based platform containing sea and weather data – for example, water levels, salinity, wind speeds, visibility and currents. The platform can be used to predict the best time for mooring and departure, with the guarantee of maximum capacity utilisation, based on the recorded water level, currents and wind conditions.
The vision is of a port where quay walls ‘talk’ with ships, containers with cranes, terminals with trains, distribution centres with trucks. These exchanges help the port to optimally utilise the available capacity. The Port of Rotterdam Authority is currently fitting assets with the required sensors. Hundreds have been installed and are being installed, giving a mass of insights and potential improvement opportunities from their readings. For example, this datadriven approach already allows dredging operations based on necessity rather than a fixed schedule as before.
All these, as we said at the start, are works in progress. Ai is in infancy. What it will do when it is grown up is anyone’s guess. Perhaps we should ask AI to tell us?